The Power of Agriculture Datasets for Machine Learning in Transforming the Farming Landscape
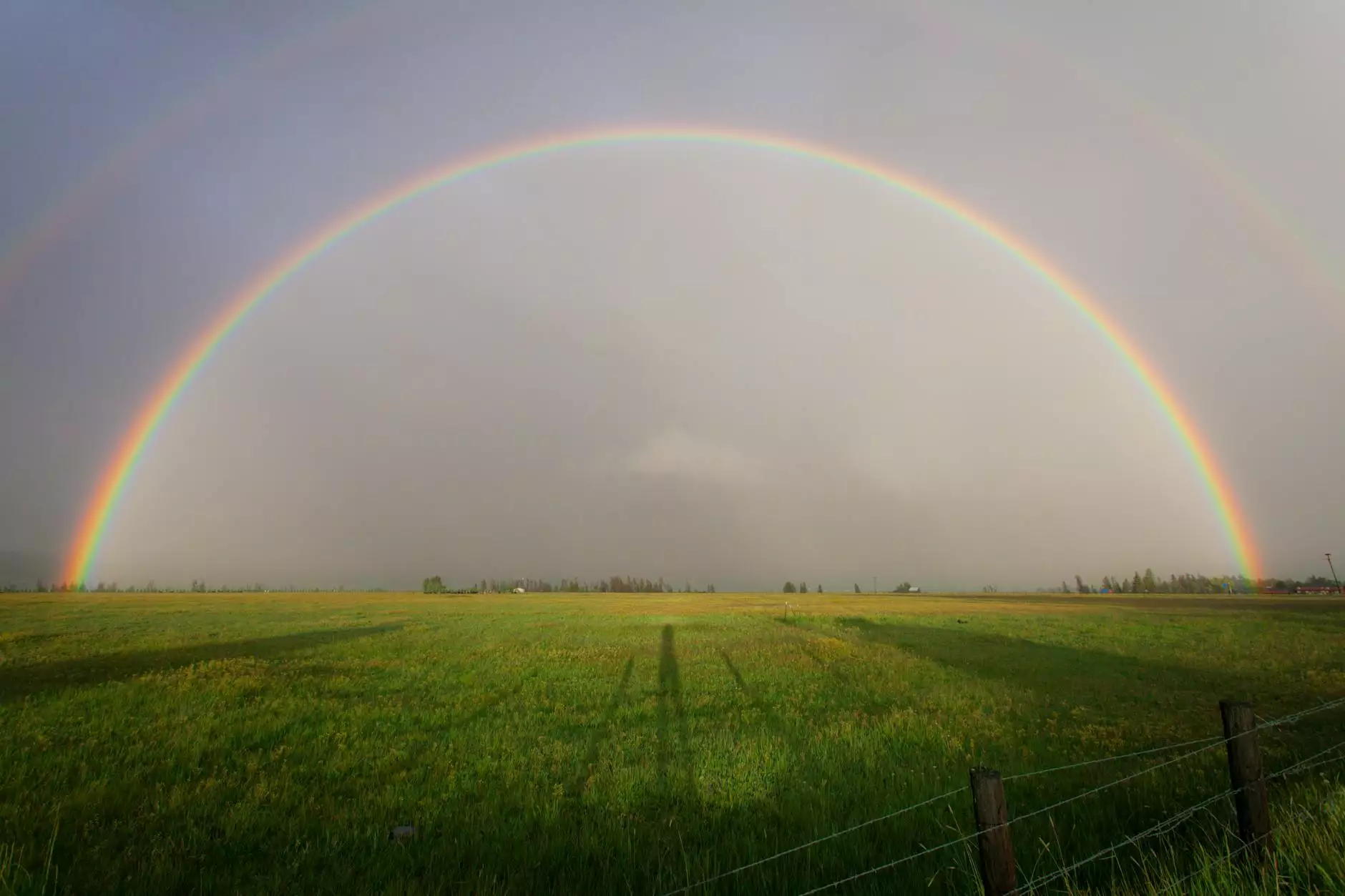
In today's digital age, the integration of machine learning into various industries has become commonplace, and agriculture is no exception. The advent of agriculture datasets for machine learning has opened up a world of possibilities, enabling farmers and agribusinesses to leverage data-driven insights for improved decision-making and operational efficiency.
Understanding Agriculture Datasets for Machine Learning
Agriculture datasets consist of various types of data that can be used to train machine learning models. These datasets may include:
- Soil Data: Information regarding soil composition, pH, moisture levels, and nutrient content.
- Weather Data: Historical and real-time data about temperature, rainfall, humidity, and other climatic factors.
- Crop Data: Details about crop yields, species characteristics, and growth patterns.
- Pest and Disease Data: Information on pest populations, disease outbreaks, and management strategies.
- Market Data: Market trends, prices, and demand analytics that affect the agricultural economy.
The Role of Machine Learning in Agriculture
Machine learning utilizes algorithms and statistical models to analyze and interpret large amounts of data, helping in predicting outcomes and making informed decisions. In agriculture, machine learning assists in:
1. Precision Farming
By utilizing agriculture datasets for machine learning, farmers can practice precision farming which involves the use of technology to monitor field variability in crops. This allows for tailored interventions based on soil conditions, crop needs, and environmental factors.
2. Crop Prediction
Machine learning models can analyze historical data to predict future crop yields based on various factors such as weather patterns and soil health. This predictive capability enables farmers to optimize inputs like water and fertilizers, thus ensuring maximum yield.
3. Pest and Disease Management
Identifying and managing pests and diseases can be extremely challenging. Machine learning algorithms can analyze patterns in data to identify early signs of crop disease or pest infestation, allowing for timely interventions that can save crops from extensive damage.
Benefits of Agriculture Datasets for Machine Learning
Utilizing agriculture datasets for machine learning comes with numerous benefits that enhance the productivity and sustainability of farming practices:
1. Improved Yield and Productivity
By leveraging data, farmers can make data-driven decisions that lead to increased crop yields. This can be achieved through optimized resource allocation and by understanding which crops are best suited for specific conditions.
2. Cost Efficiency
Machine learning can help reduce waste by optimizing the use of inputs such as fertilizers, pesticides, and water. By analyzing data, farmers can apply the right amount of resources at the right time.
3. Sustainable Farming Practices
Machine learning combined with big data helps promote sustainable agricultural practices. By predicting environmental impacts and adjusting farming techniques accordingly, farmland can be preserved for future generations.
4. Enhanced Decision-Making
Access to comprehensive datasets allows farmers and agricultural businesses to make decisions backed by data. This leads to better management strategies and reduced operational risks.
Challenges in Utilizing Agriculture Datasets for Machine Learning
While the prospects are promising, there are several challenges in harnessing agriculture datasets for machine learning:
1. Data Quality and Availability
The effectiveness of machine learning models largely depends on the quality of data. In agriculture, obtaining high-quality, diverse datasets can often be challenging. Intermittent data collection and variability can hinder the model training process.
2. Integration of Data Sources
Data in agriculture often comes from disparate sources including satellites, sensors, and field reports. Integrating these varied data streams into a coherent dataset usable by machine learning algorithms can be complex.
3. Technical Expertise
Farmers may lack the technical expertise required to implement machine learning solutions effectively. Training and support systems are crucial to bridge this gap and ensure that farmers can leverage these advanced technologies.
Future Trends in Agriculture Datasets for Machine Learning
The future of agriculture datasets and their application in machine learning holds exciting possibilities. Here are some trends to watch for:
1. Increased Use of IoT Devices
With the continued rise of the Internet of Things (IoT), farmers will increasingly utilize smart sensors that will generate real-time data on crop health, soil conditions, and environmental factors. This data can seamlessly feed into machine learning models for accurate predictions and insights.
2. Enhanced Data Sharing Platforms
As more stakeholders recognize the value of data, initiatives to share agricultural datasets will proliferate, allowing for richer datasets that can produce more robust machine learning models. Collaboration across institutions, governments, and organizations can drive innovation.
3. Development of Open-Source Tools
Open-source machine learning tools and platforms will become more prevalent, making it easier for farmers to access and utilize sophisticated algorithms without requiring extensive technical knowledge. This democratization of technology will empower farmers to adopt data-driven strategies.
Conclusion
In summary, the integration of agriculture datasets for machine learning is transforming the agricultural landscape by enhancing productivity, sustainability, and decision-making processes. As data availability increases and machine learning technologies evolve, the potential to optimize farming operations has never been greater. It is an exciting time for agriculture, where data and technology together pave the way toward a more efficient and sustainable future.
For agribusinesses and farmers to remain competitive in today’s complex market, embracing the power of data and machine learning will be imperative. With comprehensive agriculture datasets at their disposal, the agricultural industry stands on the brink of a revolution, ready to take bold steps into the future.
agriculture dataset for machine learning